27 Jan 2025
TLDR: I found ResizableChannel
in github.com/eapache/channels
and used it to dynamically change the number of goroutines running at the same time
For a CPU intensive and parallel task I needed a solution to be able to throttle it.
During the day it should simmer and use one core, not to disturb other running processes, but during the night it could use a lot more cores.
Since it was written in Go, and already used goroutines, I decided to dynamically limit the number of goroutines running at the same time.
I’m aware that number of goroutines is not necessarily the same as the number of cores used, but I’ll use them interchangeably anyway.
In my code, they were roughly the same.
Golang’s recommended way is to use a limit on a group.
This is challenging, because the documentation says you can (should) not change the limit while goroutines are running.
If you want to change it, you should wait until all concurrent goroutines are done, change it, and continue with the new limit.
Depending on your use case this is probably not ideal.
A second pattern Go programs often use is a channel with a limit; a buffered channel.
By limiting the number of slots in the channel, and getting them out once the goroutine is done, it automatically limits the number of concurrent goroutines.
On Go’s default chan
it’s not possible to change the size, however.
I’ve slightly modified the example from the Go Wiki.
The below example shows how a buffered channel would typically limit the number of running goroutines.
package main
import (
"runtime"
)
var goroutineLimit = make(chan struct{}, runtime.NumCPU())
func Serve(queue chan *Request) {
for {
goroutineLimit <- struct{}{} // Block until there's capacity
req := <-queue
go handle(req)
}
}
func handle(r *Request) {
process(r) // May take a long time & use a lot of memory or CPU
<-goroutineLimit // Done; enable next request to run.
}
This is almost what I need. If only the Go channel was resizable…
What felt like a naive approach: googling for a Go channel alternative, but make it resizable, actually yielded results.
The library github.com/eapache/channels
offers just that, a ResizableChannel
implementation.
While this library says it’s no longer maintained, I didn’t encounter any issues using the current Go runtime.
It builds, and the built executables didn’t panic.
Go code can sit untouched for years and still work fine.
Below are the changes that you should make to switch to ResizableChannel
:
package main
import (
+ "github.com/eapache/channels"
"runtime"
)
-var goroutineLimit = make(chan struct{}, runtime.NumCPU())
+var goroutineLimit = channels.NewResizableChannel()
func Serve(queue chan *Request) {
+ goroutineLimit.Resize(channels.BufferCap(runtime.NumCPU())) // Initial channel size
for {
- goroutineLimit <- struct{}{} // Block until there's capacity
+ goroutineLimit.In() <- struct{}{} // Block until there's capacity
req := <-queue
go handle(req) // Don't wait for handle to finish.
}
}
func handle(r *Request) {
- process(r) // May take a long time & use a lot of memory or CPU
- <-goroutineLimit // Done; enable next request to run.
+ process(r) // May take a long time & use a lot of memory or CPU
+ <-goroutineLimit.Out() // Done; enable next request to run.
}
Note that the diff above doesn’t have a way of actually changing the channel size yet.
How you communicate that the number of concurrent goroutines should change depends on your use case.
You could consider doing it with gRPC, but it’s also possible to run a simple webserver that accepts a number as GET parameter.
Here’s a server that you could start early in your program that would do the latter:
go func() {
log.Println("Starting web server on port 8080...")
http.HandleFunc("/resize", func(w http.ResponseWriter, r *http.Request) {
var newSize int
if r.Method != http.MethodGet {
http.Error(w, "Invalid request method", http.StatusMethodNotAllowed)
return
}
sizeParam := r.URL.Query().Get("new_size")
if sizeParam == "" {
return
}
newSize, err := strconv.Atoi(sizeParam)
if err != nil || newSize <= 0 {
http.Error(w, "Invalid 'new_size' parameter", http.StatusBadRequest)
return
}
goroutineLimit.Resize(channels.BufferCap(newSize))
// log.Printf("Resized goroutine limit to %d", newSize)
w.WriteHeader(http.StatusOK)
})
if err := http.ListenAndServe(":8080", nil); err != nil {
log.Fatalf("Failed to start web server: %v", err)
}
}()
After creating this solution to dynamically limit the number of concurrent goroutines, I read an interesting discussion about using weighted Semaphores to achieve more or less the same.
There has also been an effort to make Golang’s own weighted Semaphore
implementation resizable, but this has not been merged.
In the end this feature was not included in the default Semaphore implementation.
The discussion references that the quotapool
package by cockroachdb
might be a solution.
I didn’t look into that too much, since it is a bit more complicated.
It can deal with heterogeneous work units, and I simply don’t need that.
Each of my tasks consumes one core fully (but briefly), and always takes about the same time.
I like this solution because it only requires a few lines of changes in existing code.
(Plus some logic to change the capacity of the channel, but how to handle that will vary on your situation).
It could still be better in one way:
if the resizable channel actually has meaningful variables in it, instead of empty struct
s.
By actually communicating useful items it would function as a “proper” channel instead of as a locking hack.
14 Nov 2024
TLDR: Stripping metadata from a Microsoft Office file can be done with a single 7-zip command:
7z d file.docx "docProps/*"
When you save a docx file, it can contain metadata like the author, title, and comments.
On StackOverflow, a user asked how to remove this metadata.
One of the answers was useful to me, but it didn’t strip all the metadata and replaced some fields with the wrong data type.
It uses the python-docx
library, so you would need to pip install
that first.
This library knows that a docx is a zip in a trenchcoat, and can modify the XML in this zip inplace.
I’ve modified the answer to set all metadata fields to an empty or null-ish value.
from datetime import datetime
from docx import Document
document_path = 'example.docx'
# Strip metadata
document = Document(document_path)
metadata_fields = {
"author": str,
"category": str,
"comments": str,
"content_status": str,
"created": lambda: datetime(2000, 1, 1),
"identifier": str,
"keywords": str,
"language": str,
"last_modified_by": str,
"last_printed": lambda: datetime(2000, 1, 1),
"modified": lambda: datetime(2000, 1, 1),
"revision": int,
"subject": str,
"title": str,
"version": str,
}
for meta_field, factory in metadata_fields.items():
setattr(document.core_properties, meta_field, factory())
# Save the document
document.save(document_path)
The snippet above was my preferred way of removing metadata for quite some time.
However, when creating a document with WordPad I noticed it didn’t even create a docProps
directory at all.
So it seems this entire directory is optional for the specification, and therefore optional to be a valid docx file.
That means we don’t have to bother with changing the XML in the file at all.
With a single command we can delete all metadata (including fields like edit duration of the document).
7z d file.docx "docProps/*"
On Windows you the 7-zip command line tool can be named 7z.exe
instead, and it might not be on your PATH
.
This command also works for other Microsoft Office tools like Excel or Powerpoint:
7z d file.xlsx "docProps/*"
7z d file.pptx "docProps/*"
Excel files can contain edit history, which may contain some data you might want to delete too.
This is especially important if you’re trying to commit academic fraud.
So if you want to be certain that some information is no longer in your file, extract your file and grep
around for it.
After you’ve done this, you might be tempted to check if you have not accidentally corrupted the file.
Note that if you do that, and you accidentally save it, you have re-introduced the docProps
directory.
As a final warning, know that 7zip will not ask if you’re sure about the command, so you may lose work.
24 Jul 2024
If you’re used to the Dvorak keyboard layout, you might have a hard time using the GPD Pocket 3.
In a QWERTY layout the ;
is to the right of the L.
GPD moved this to the right of the spacebar on the Pocket 3.
The location of the ;
is used for the letter s
in the Dvorak layout.
That means a very frequently used letter is no longer on the home row, defeating the purpose of the Dvorak layout quite
a bit.
This was done on Ubuntu 24.04. Your experience on other Linux distributions might vary.
I found the following remapping quite easy to get used to:
- Enter becomes the letter S
- Backspace is the new Enter
- Delete is now backspace
- Shift + Delete is the delete action
(Although not related to the GPD Pocket 3 specifically I also remapped Caps Lock to Control.)
Note that these changes are applied on top of simply switching to the Dvorak layout:
Original layout |
Changes on top of setting the layout to Dvorak |
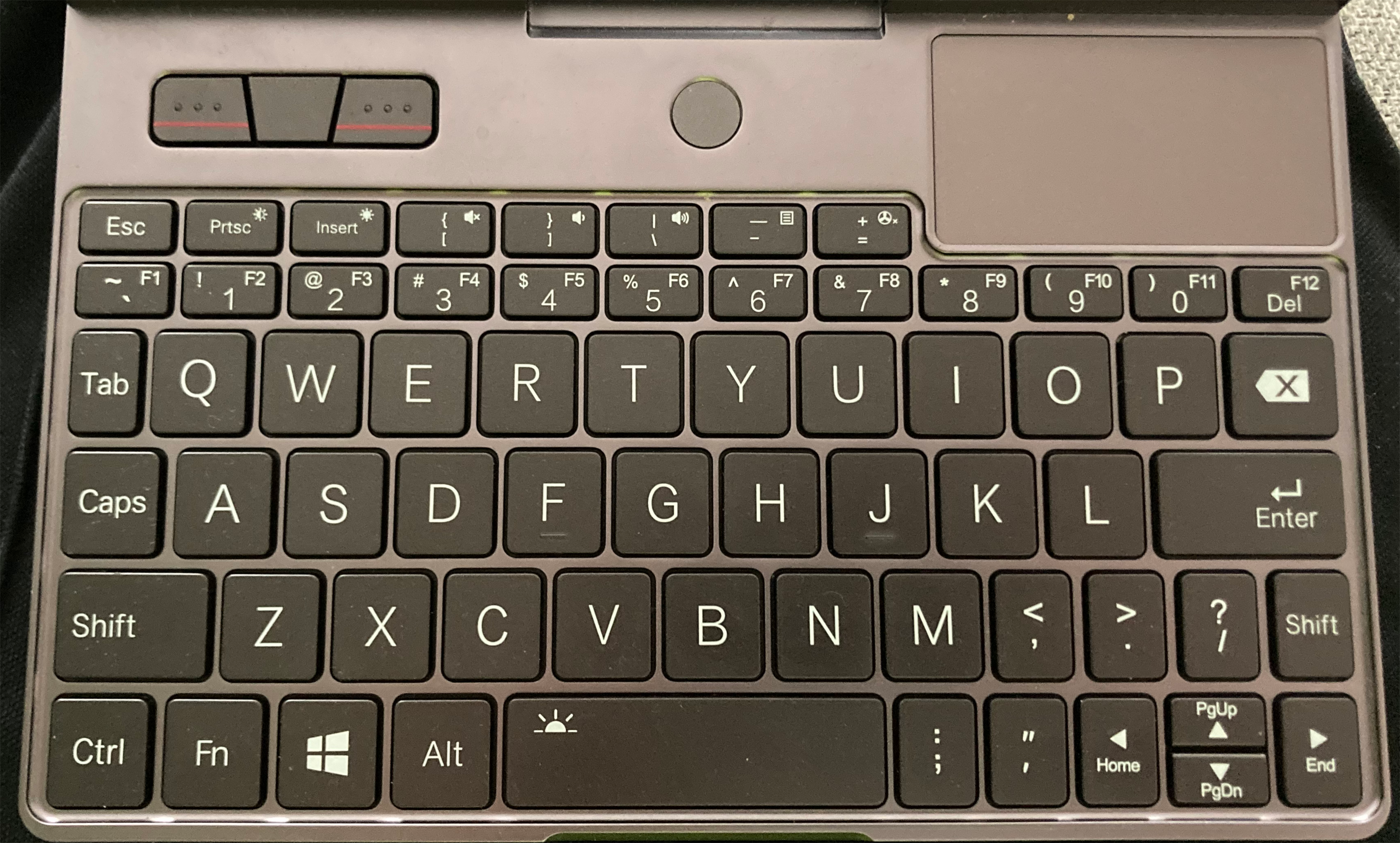 |
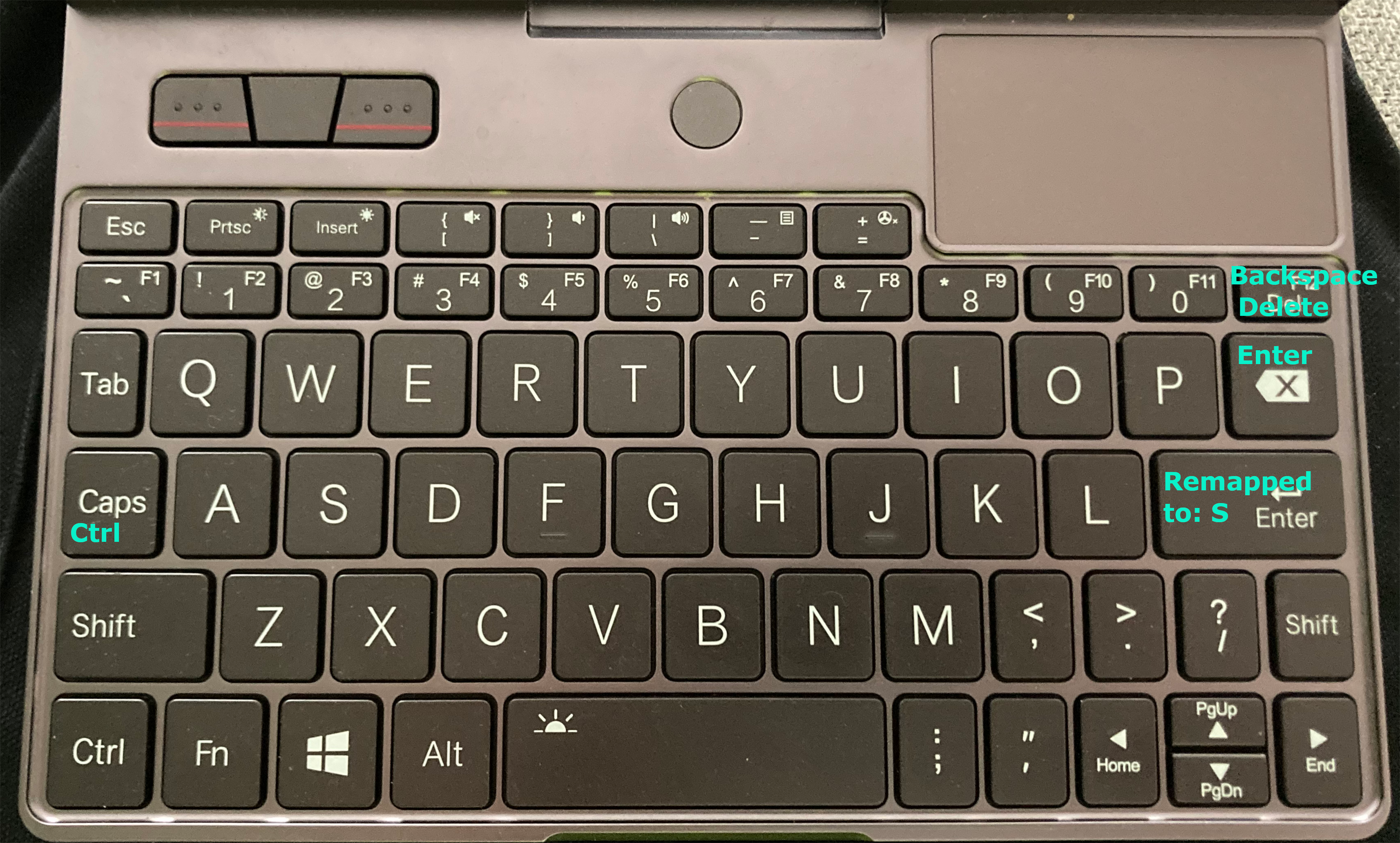 |
In practice this results in the following changes to the file /usr/share/X11/xkb/symbols/pc
config flle.
--- pc.bak 2024-05-14 08:04:51.859190653 +0200
+++ pc 2024-05-14 08:54:16.902085341 +0200
@@ -4,11 +4,11 @@
key <ESC> {[ Escape ]};
key <TAB> {[ Tab, ISO_Left_Tab ]};
- key <CAPS> {[ Caps_Lock ]};
+ key <CAPS> {[ Control_R ]};
- key <BKSP> {[ BackSpace, BackSpace ]};
+ key <BKSP> {[ Return ]};
key <BKSL> {[ backslash, bar ]};
- key <RTRN> {[ Return ]};
+ key <RTRN> {[ s, S ]};
// The extra key on many European keyboards:
key <LSGT> {[ less, greater, bar, brokenbar ]};
@@ -59,7 +59,7 @@
key <PAUS> {[ Pause, Break ], type="PC_CONTROL_LEVEL2" };
key <INS> {[ Insert ]};
- key <DELE> {[ Delete ]};
+ key <DELE> {[ BackSpace, Delete ]};
key <HOME> {[ Home ]};
key <END> {[ End ]};
key <PGUP> {[ Prior ]};
Note that this configuration only takes effect in X.
Your remap does not apply yet for example when booting and unlocking the full disk encryption.
The configuration change also does not survive some updates, so you might need to restore it from time to time.
It might be better to achieve the same results with xmodmap
.
I have not yet done this successfully though.
If my current solution of modifying the XKB config gives problems, I might try xmodmap
next.
27 May 2024
Most common implementations of the HDBSCAN clustering algorithm don’t have a predict
method.
There are some fundamental reasons that many implementations don’t have it.
These reasons mostly boil down to the following: Introducing a new data point might change the clustering.
But depending on your use case, it can be valid to want or need a predict
method anyway.
For example you could be using scikit learn pipelines, that expect a predict
method for the last transformer.
Or you might be sure that the samples you want to cluster look a lot like your training data.
Maybe the samples you offer to the predict
method are even drawn from the data you fitted the clusterer on.
For all those use cases the people maintaining the hdbscan
package have invented the approximate_predict
utility.
We could move the functionality in this method to the predict
method of the HDBSCAN
class.
Because not all implementations have something like this method, here I’ll assume the hdbscan
package is being used.
The code below shows how to monkey patch a predict method on an instance of HDBSCAN
:
from hdbscan import HDBSCAN
from hdbscan.prediction import approximate_predict
from sklearn.datasets import make_blobs
# Generate some sample data to cluster
blobs, _ = make_blobs(
n_samples=750,
centers=[[1, 1], [-1, -1], [1.5, -1.5]],
cluster_std=[0.4, 0.1, 0.75],
random_state=0,
)
# Instantiate HDBSCAN with prediction_data=True so approximate_predict will work
clusterer = HDBSCAN(prediction_data=True)
# Monkey patch the approximate_predict method as predict method on the instance
clusterer.predict = lambda x: approximate_predict(clusterer, x)[0]
# Now the predict method is available
fitted = list(clusterer.fit_predict(blobs))
predicted = list(clusterer.predict(blobs))
assert fitted == predicted
Alternatively you could subclass the HDBSCAN
class.
Since scikit learn does not accept varargs for init methods of estimators, this gets verbose:
from hdbscan import HDBSCAN
from hdbscan.prediction import approximate_predict
from joblib import Memory
class HDBSCANWithPredict(HDBSCAN):
def __init__(self,
min_cluster_size=5,
min_samples=None,
cluster_selection_epsilon=0.0,
max_cluster_size=0,
metric="euclidean",
alpha=1.0,
p=None,
algorithm="best",
leaf_size=40,
memory=Memory(None, verbose=0),
approx_min_span_tree=True,
gen_min_span_tree=False,
core_dist_n_jobs=4,
cluster_selection_method="eom",
allow_single_cluster=False,
prediction_data=True, # changed from the reference implementation
match_reference_implementation=False,
**kwargs):
super().__init__(min_cluster_size=min_cluster_size,
min_samples=min_samples,
cluster_selection_epsilon=cluster_selection_epsilon,
max_cluster_size=max_cluster_size,
metric=metric,
alpha=alpha,
p=p,
algorithm=algorithm,
leaf_size=leaf_size,
memory=memory,
approx_min_span_tree=approx_min_span_tree,
gen_min_span_tree=gen_min_span_tree,
core_dist_n_jobs=core_dist_n_jobs,
cluster_selection_method=cluster_selection_method,
allow_single_cluster=allow_single_cluster,
prediction_data=prediction_data,
match_reference_implementation=match_reference_implementation,
**kwargs)
def predict(self, points_to_predict):
return approximate_predict(self, points_to_predict=points_to_predict)[0]
Whether you choose the monkey-patching or the subclassing approach, you now have a predict
method available.
Even though inference is now possible for new points, it’s best to keep monitoring the performance of this clusterer.
Out-of-cluster samples can be recognised by a label of -1
.
A dead giveaway that your trained clusterer is no longer appropriate is when the fraction of out-of-cluster samples is:
- a lot higher than in the training set
- rising over time because of changing data
If the inference is no longer acceptable you should re-fit HDBSCAN.
02 Apr 2024
Streamlit has an API to add custom HTML to your document, but the added HTML will be nested in an iframe.
Since the policies on this iframe are quite relaxed, you can use window.parent
to escape to the parent document.
This can be useful if you want access to elements on the top-level DOM.
Using the following code, the HTML added will lift itself out of its containing iframe:
from streamlit.components.v1 import html
html_contents = """
<script id="extractorScript">
let currentScript = document.getElementById('extractorScript');
window.parent.document.querySelector('iframe').insertAdjacentElement(currentScript.nextSibling);
// window.parent.document.querySelector('iframe').remove();
</script>
<div>
<h1>Test contents</h1>
<p>The HTML contents that you want to move out of its iframe</p>
</div>
"""
html(html_contents)
Prepending the contents of the script
tag to your HTML tag (a div
in this case) allows it to find the content that needs to be lifted with the .nextSibling
attribute.
If you’re planning to use this code snippet more than one time, you will have multiple iframe
elements.
In that case it’s wise to come up with a more specific CSS selector than 'iframe'
.